Category-Based Prejudice
Properties of a population are not properties of individuals, and categorization is arbitrary. Therefore, category-based prejudice is more costly, less accurate, and more ambiguous than direct measurement.
Table of Contents
Terminology
This article discusses the fallacies associated with judging an individual based on how the individual is categorized. The word “prejudice” can be used to name this practice. However, the word “prejudice” in the English language can be interpreted either too broadly or too narrowly.
For instance, the first definition for “prejudice” in the American Heritage Dictionary of the English Language consists of “the act or state of holding unreasonable preconceived judgments or convictions.” (Pickett 2018) This first definition is more broad than how “prejudice” is used in this article; there are many different kinds of prejudice so construed, beyond the specific kind discussed in this article.
The second definition for “prejudice” in the American Heritage Dictionary of the English Language is “irrational suspicion or hatred of a particular social group, such as a race or the adherents of a religion.” (Pickett 2018) This is similar to how the word “bigotry” is used in contemporary discourse.
This second definition is more narrow than how “prejudice” is used in this article in at least two ways. First, this definition applies specifically to judgments about human beings, whereas the criticisms discussed here apply equally well when reasoning about rocks, single-celled organisms, music, countries, or anything else that can be categorized. Second, this definition construes “prejudice” as referring to judgments that are undesirable, whereas the way “prejudice” is used in this article can refer to judgments about desirable traits, as well.
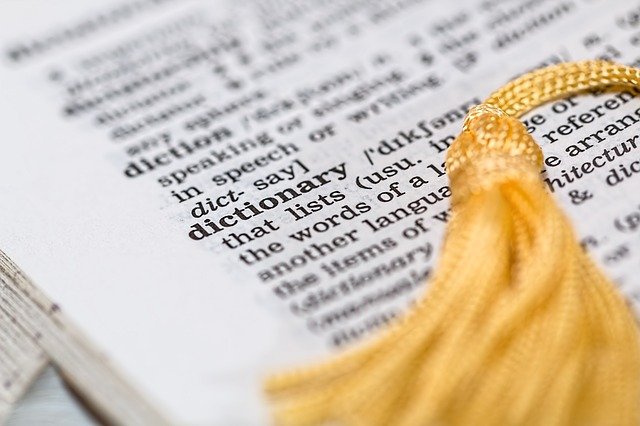
Because the English language lacks a word with the precise scope used by this article, the phrase “category-based prejudice” is used.
Analytical versus Moral Criticism
The criticisms this article puts forth of category-based prejudice are entirely with regard to the flaws such prejudice imparts on attempts to understand the world. Much can be written about the moral aspects of category-based prejudice targeting human beings in particular, but writing about the evils of bigotry is not the purpose this article and is left to works of social science or history. Instead, this article focuses on criticisms of category-based prejudice as a form of reasoning, regardless of the consequences of such thinking.
Thus, the criticisms articulated here are independent of the moral dimensions of category-based prejudice. This article puts forward the thesis that category-based prejudice is always a flawed way of thinking, even if there does not appear to be anything morally objectionable about it. Indeed, even if one judges an instance of category-based prejudice as morally justified, the fallacies in reasoning discussed in this article persist.
Abstract Example
Before the particular flaws of category-based prejudice are examined, two general principles of categorization are discussed by way of an abstract example.
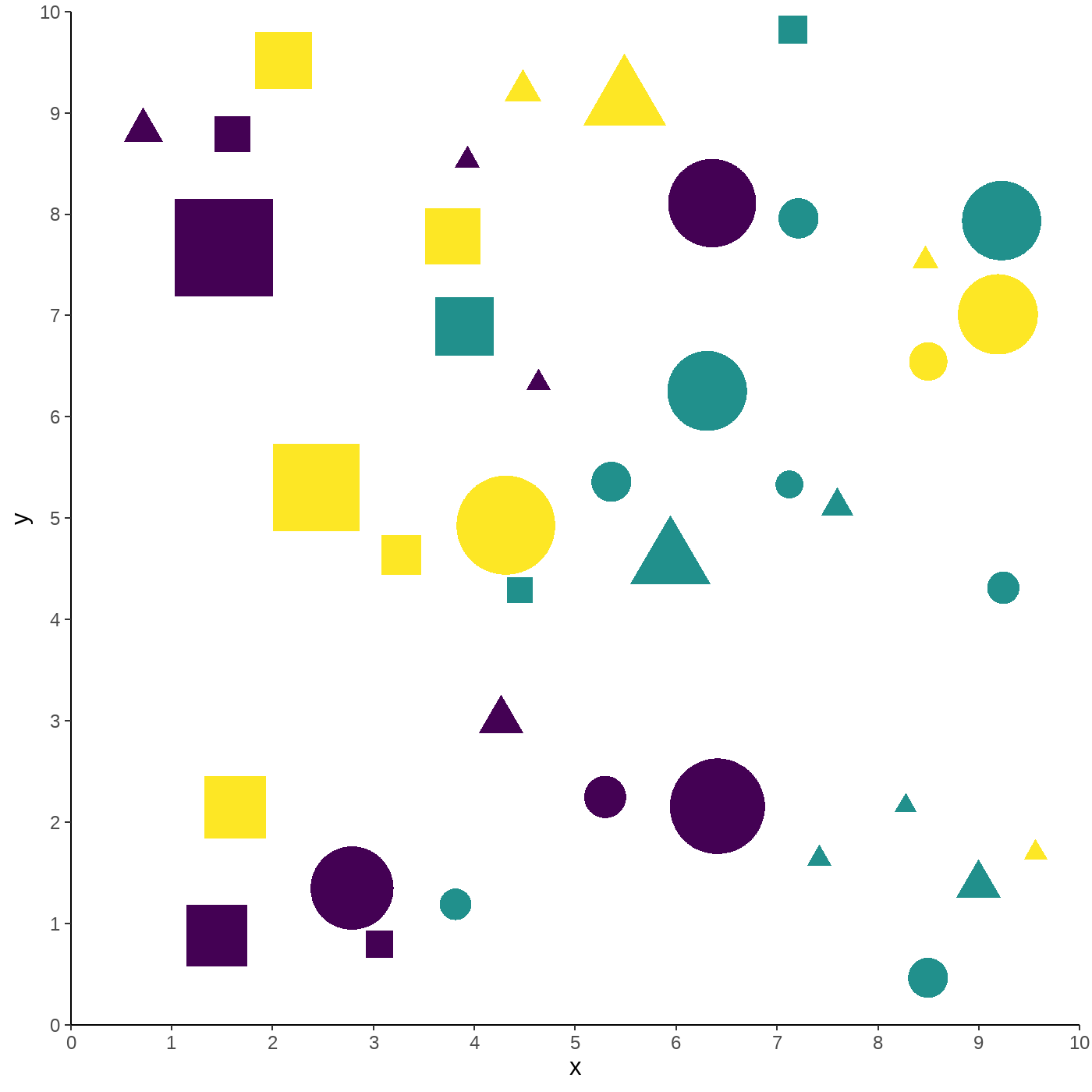
Figure 1: Geometric figures of various colors, sizes, and shapes, laid out on a two-dimensional plane.
The principles are illustrated by the population in Figure 1, which consists of 40 individual geometric figures. The shape, color, position, and size of each geometric figure have been randomly selected according to specific probability distributions.1
Properties of a Population Are Not Properties of Individuals
Table 1: Diameter, location, and color information for the circles portrayed in Figure 1.
Diameter | x | y | Color |
---|---|---|---|
0.28 | 7.12 | 5.33 | cyan |
0.31 | 3.81 | 1.19 | cyan |
0.32 | 9.24 | 4.31 | cyan |
0.38 | 8.50 | 6.55 | yellow |
0.39 | 5.36 | 5.36 | cyan |
0.39 | 8.50 | 0.46 | cyan |
0.40 | 7.21 | 7.96 | cyan |
0.42 | 5.30 | 2.25 | purple |
0.79 | 9.23 | 7.94 | cyan |
0.79 | 6.31 | 6.26 | cyan |
0.79 | 9.19 | 7.01 | yellow |
0.82 | 2.79 | 1.35 | purple |
0.87 | 6.36 | 8.11 | purple |
0.94 | 6.41 | 2.16 | purple |
0.98 | 4.31 | 4.93 | yellow |
Table 1 lists the diameter dimensions for all 15 circles in Figure 1, sorted from smallest to largest and rounded to the hundredths. The arithmetic mean of all these diameters is 0.59. Thus, the average circle diameter is 0.59. However, none of the actual circles in Figure 1 have a diameter of 0.59.2
This illustrates the principle that properties of a population are not properties of individuals. Descriptive statistics like the arithmetic mean of a variable describe properties of a population. They do not describe any property of an individual, even if that individual is categorized as part of the population in question. While it might be common to use language such as “the average circle has diameter 0.59,” the average circle does not actually exist. It is an unfortunate figure of speech.
While this may seem obvious when applied to a very visual example such as geometric figures, it is important to remember this principle whenever thinking about populations of anything. There is no entity named by “the average person.” The average person does not exist.
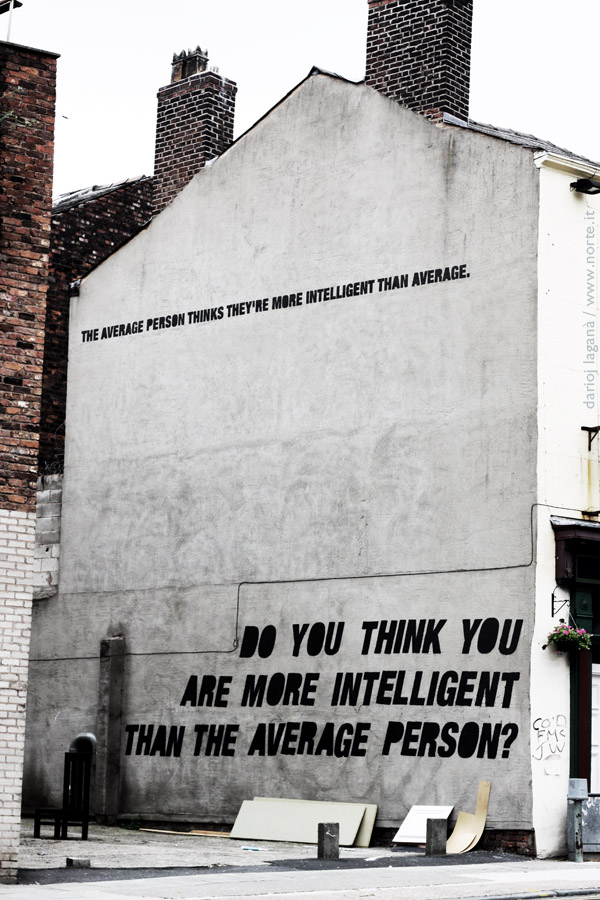
This principle applies not just to averages, but to any property of a population. For instance, of the geometric figures in Figure 1, 40% are cyan, 30% are purple, and 30% are yellow, but there is not a single geometric figure that is 40% cyan, 30% purple, and 30% yellow; all of the figures are exclusively one color. Thus, this principle applies not just to averages, but also to proportions. Indeed, it applies to any property of a population.
Categorization Is Arbitrary
The reason why properties of a population are not properties of individuals is that categorization is arbitrary. The act of categorization is the creation of a convention that abstracts away details and simplifies thinking about numerous individuals simultaneously. There is nothing wrong with this practice. Attempting to think about large numbers of individuals simultaneously would be overly complicated without such simplification.
However, it is important to remember that any convention done one way could be also be done a different way. Individuals are only included as members of a population because someone construes them to be, whether this is through the formal creation of rules for what individuals get included in which categories or through an ad hoc process in which someone manually categorizes individuals as they are encountered.
Regardless of how it is done, the choice of how to do the categorization in the first place is itself arbitrary. Any given individual categorized one way could have been categorized a different way under a different set of rules or with a different judge. Because the categorization itself is arbitrary, the act of categorization, while potentially useful for further analysis, is not itself informative.
Choice of Variable for Categorization
For instance, suppose that a new cyan square will be added to Figure 1, using the same probability distributions used to generate the existing geometric figures. What expectations can be had about the position of this new cyan square?
Looking at the shapes farthest to the right, there are no squares, and looking at the shapes farthest to the left, there are mostly squares. There thus appears to be a trend in which squares tend to be farther to the left in Figure 1. Indeed, while the average x position for all figures is 5.39, the average x position for squares specifically is 3.03. Category-based prejudice should therefore expect a square to be positioned more to the left of the two-dimensional plane in Figure 1.
On the other hand, looking at the colors farthest to the right, there are mostly cyan figures, and looking at the shapes farthest to the left, there are no cyan figures. There thus also appears to be a trend in which cyan figures tend to be farther to the right in Figure 1. Indeed, the average x position for cyan figures specifically is 6.91. Category-based prejudice should therefore expect a new cyan figure to be positioned more to the right of the two-dimensional plane in Figure 1.
In this example, category-based prejudice leads to two contradictory expectations, depending on the choice of categorization. If the new cyan square is categorized according to shape, category-based prejudice expects it on the left, but if the new cyan square is categorized according to color, category-based prejudice expects it on the right. Because categorization is arbitrary in the choice of variable to use for categorization, category-based prejudice is arbitrary in ambiguous cases such as these. This phenomenon is discussed further later in this article in the context of a more concrete example.
Choice of Levels of a Variable
Even after the choice of variable to be used for categorization is settled, the choice of the number of possible category options, sometimes called the “levels” of a categorical variable, is arbitrary. For instance, if the geometric figures in Figure 1 are to be categorized by size, the number of levels to use for this categorization could be just two (e.g., “small” or “large”), three (e.g., “small,” “medium,” or “large), or any arbitrary number of levels.
Furthermore, the choice of where to set the boundaries in between the levels of a categorical variable is arbitrary, and this choice especially can be abused.
For instance, if the geometric figures in Figure 1 are categorized into “small” and “large” levels based on whether the area of the figure is less than or greater than 0.45, this results in a mean x position of 5.39 for small figures and a mean x position of 5.39 for large figures. This has the result of making it appear that the size of a figure does not make the figure any more likely to appear on the left or the right of the two-dimensional plane. When dividing a numerical variable into discrete levels, values such as 0.45 are often called the “cut point.”
However, if instead of 0.45, a cut point of 0.50 is used to categorize the figures into “small” and “large” levels, this leads to a mean x position of 3.97 for large figures and a mean x position of 5.65 for small figures. This has the result of making it appear that larger figures are more likely to appear on the left of the two-dimensional plane.
Even though the same exact underlying population is used to calculate both sets of statistics, the choice of a different cut point lead to different results. Because the choice of cut point is arbitrary, this could be used deceptively. Those who set out to “tell a story with the data” would choose the cut point that best fits the story they wish to tell.
Concrete Example: Gender Prejudice in Hiring
In order to illustrate the main flaws with category-based prejudice, this article turns from the abstract to more concrete examples. Suppose that a hiring manager at a company is considering what to look for in the next employee to be hired and is told the next hire should be a woman because the team needs more empathy and women are more empathic. Alternatively, the manager is told to hire a man because the team needs more math ability and men are good at math.
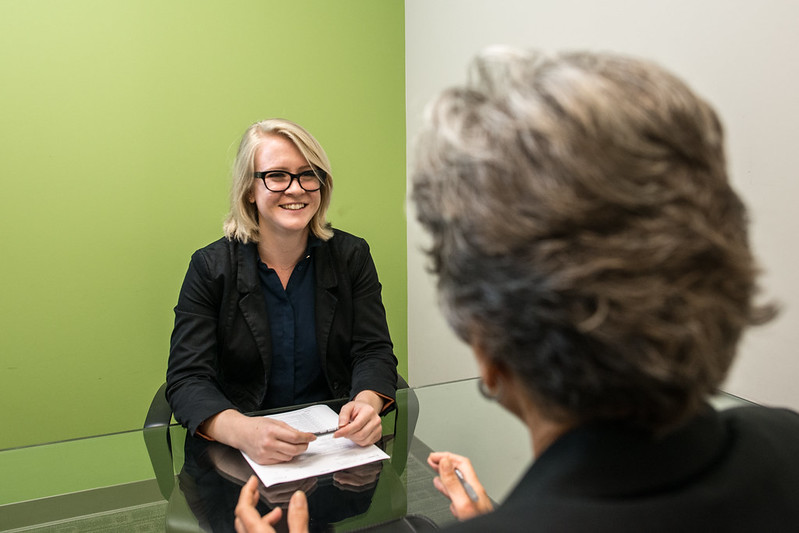
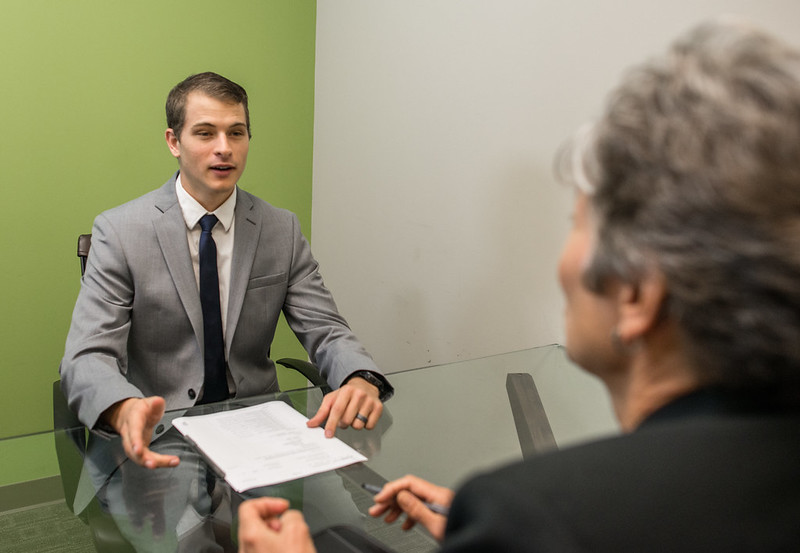
Further suppose in either scenario, the hiring manager has narrowed the search for a new employee to two people, one a woman and one a man. These two prospects seem equally qualified in other respects besides math ability or empathy, and all that remains is to choose between the two prospective employees.
Direct Measurement
How could propositions such as whether someone is “good at math” or “more empathic” be evaluated?
Math is a broad subject, and the skill sets for doing arithmetic quickly by hand, for doing university physics problems, or for proving mathematical theorems are all quite different. Those who want more math proficiency are best served to identify exactly what kind of math skills are needed. In so doing, the appropriate test for these math skills might be identified.
With regard to traits such as empathy, psychologists often spend large amounts of time and effort attempting to devise a test that is reliable (i.e., self-consistent and consistent across various contexts) and valid (i.e., actually measuring what it is supposed to measure). There are various schools of thought and approaches to development of such tests, but overall the goals of these approaches are similar: to devise a method for measuring a psychological property.
What both of these propositions presuppose, then, is that there is some instrument of measurement by which the relevant math skills or empathy traits can be discerned. Otherwise, the propositions are uninformative, pointless speech.
This raises the question: why not apply the instrument of measurement to the job applicants directly?
Category-based prejudice involves two steps. The first step is to arrive at more general propositions such as “women are more empathic” or “men are better at math.” The second step is to apply the more general proposition to the specific individuals being considered, such as the man and the woman being considered for employment. Invoking knowledge about population attributes when judging individuals thus adds a layer of indirection to the evaluation of specific individuals.
In the example scenarios, the hiring manager could simply give the two prospective employees the instrument of measurement, determine which is better than the other at empathy or at math, and hire that individual. This can be termed the “direct measurement” approach.
The category-based prejudice approach is inferior to the direct measurement approach in at least three ways:
- arriving at a more general proposition such as “women are more empathic” or “men are better at math” is more costly than directly measuring the individuals being considered,
- applying a more general proposition such as “women are more empathic” or “men are better at math” to the specific individuals is probabilistic at best and thus a weaker conclusion than directly measuring the individuals being considered, and
- the choice of variable used to judge an individual using category-based prejudice is arbitrary, which can lead to ambiguous judgments.
Additional Cost of Generalization
In order to arrive at a more general conclusion about “men” or “women,” several steps need to occur. First, a more precise target population needs to be identified. This might be all the men or women in a certain place and time frame, or perhaps all job applicants to a company. Once the target population is identified, a sampling frame must be devised that can be used to identify each individual in the target population and that allows the target population to be sampled. Once that is accomplished, a representative sample can be selected from the target population.3 The instrument of measurement can then be applied to each individual in the representative sample, and statistical analysis can done to infer estimates of the relevant values in the target population. Then, the hiring manager would know the relative math abilities or empathy levels of men and women in the population of interest.
This generalization adds additional costs to a judgment: the cost of sampling and the cost of administering the instrument of measurement to every individual in a sample large enough for statistical inference. The exact magnitude of these additional costs vary depending on the details of their circumstances.
In large surveys intended to be nationally representative, the cost of sampling can be the largest cost in the enterprise. When targeting smaller populations, such as the customers of a company, there may be preexisting records from which it is straightforward to derive a sampling frame, so the cost of sampling would be relatively small.
The cost of measuring every individual in a representative sample depends, of course, on the sample size. Sample size in turn depends on the variance in the population of the variable of interest, on the minimally detectable difference in the variable that is desired, and on the desired statistical power.4
While the exact amount of these costs might vary, they are costs that are incurred whenever a general conclusion is sought, but not costs incurred in the direct measurement approach.
Stereotypes
Of course, in practice category-based prejudice is often done based not on a valid generalization, but on little more than stereotypes stemming from anecdotes or selection bias. In these cases, there is not additional cost entailed in establishing a more general proposition, because a more general proposition has not actually been established. Thus, when appealing to stereotypes, using the category-based prejudice approach is not more costly compared to the direct measurement approach, but these cases are based entirely on fallacies and thus are worthless.
Preexisting Studies
It might sometimes be the case that the cost of arriving at a more general proposition such as “women are more empathic” or “men are better at math” is a sunk cost, when such general results have already been established by previous work. These cases do not entail additional cost for the category-based prejudice approach over the direct measurement approach, but the other criticisms described in this article remain.
Furthermore, care must be taken to discern whether the previously established results are indeed applicable. Behavioral and social science experiments do not generalize to a larger population when they are based on self-selected samples, such as the samples of 20 or 40 undergraduate student volunteers at the researchers’ university, so often discussed in the academic literature. Opinion polls done by magazines or web sites that are based on a sample of volunteers also do not generalize.
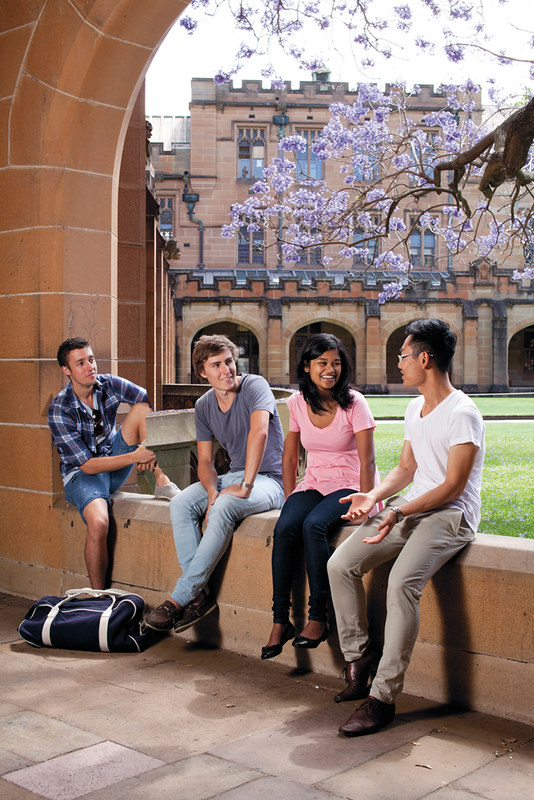
In studies that do generalize to a larger population, the sampled population of the study might not be the population of interest for the judgment being made. For instance, the hiring manager in the example scenarios is concerned with empathy or math skills in the population of job applicants to a specific company. Results discovered about the math skills of fifth graders in Idaho or the empathy skills of therapists in Massachusetts are likely not relevant for judging job applicants to the company.5 If the sampled population of a study does not contain the population of interest, attempting to apply the study’s results to the population of interest is an extrapolation outside the scope of inference of the study, which is fallacious.
Probabilistic Results
Suppose that previous work has established the distribution of empathy scores and math scores in the population of job applicants to the hiring manager’s company. If this work is based on sound statistical inference and the sampled population in this research is indeed the population of interest, then the cost of arriving at a more general proposition has already been paid.
However, the conclusion that the hiring manager can reach when applying this information to the two remaining prospective employees is a weaker conclusion than can be reached with the direct measurement approach.
Even if it is the case that the mean empathy score of women is higher than that of men among job applicants or the mean math score of men is higher than that of women among job applicants, this is not directly relevant to the hiring manager’s decision. As seen previously, properties of populations are not properties of individuals. What the hiring manager wants to know is whether the specific woman being considered has a higher empathy score than the man being considered or whether the specific man being considered has a higher math score than the women being considered.
What is relevant for the hiring manager’s decision is what has come to be called the “probability of superiority.” The probability of superiority for the empathy question is the probability that a randomly selected woman from the population of job applicants would have a higher empathy score than a randomly selected man from the population of job applicants. This requires knowledge of the probability density of the two distributions, which the supposition at the beginning of this section assumes is available.6
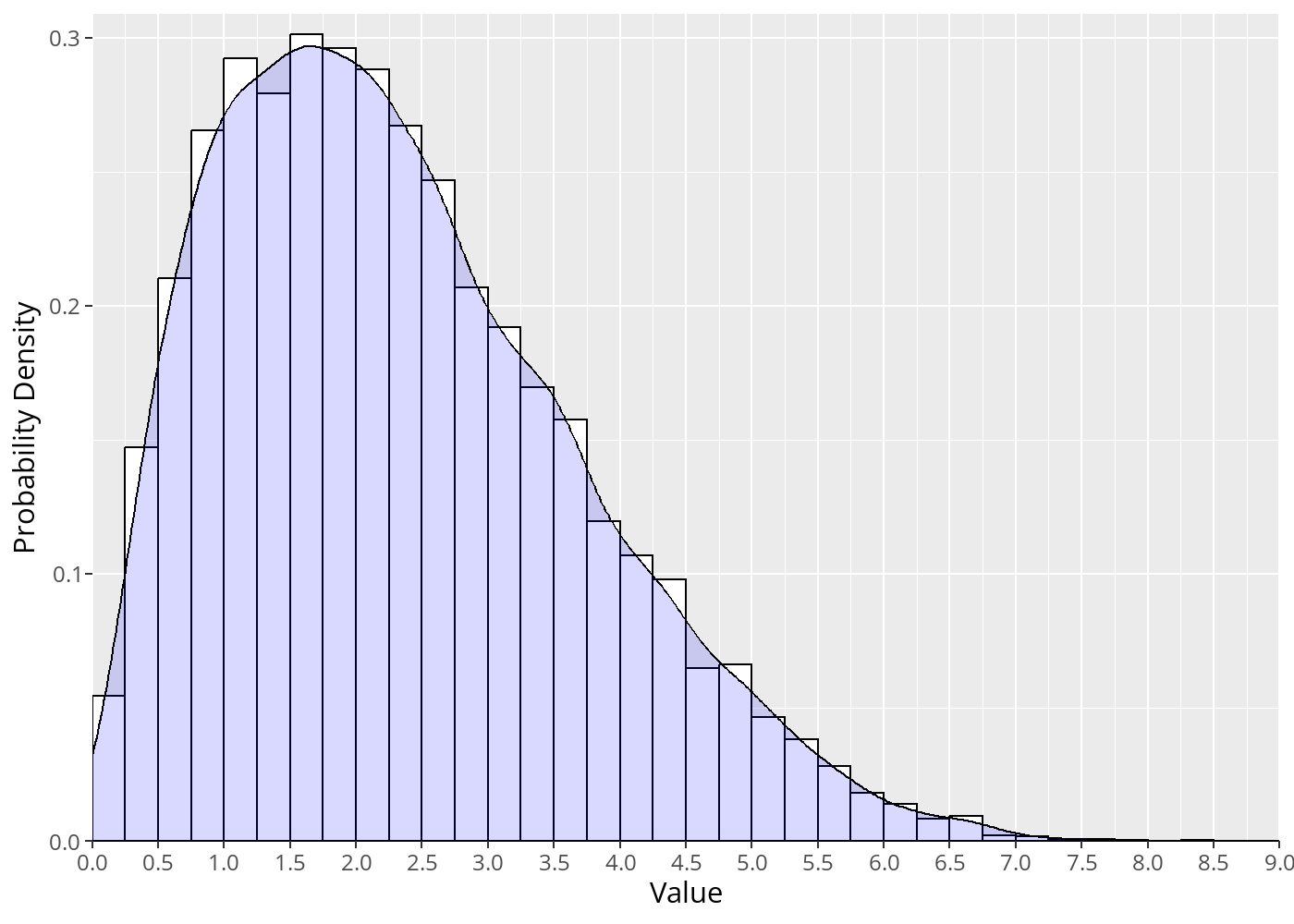
Figure 2: A histogram with an estimate of a probability density superimposed upon it. Probability densities are often represented by such plots. The x axis represents possible values of a random variable, and the y axis represents the probability of getting the values on the x axis. This random variable appears to range from 0 to about 8, to be skewed right, and to have a mode around 1.5.
Using this information, the hiring manager could calculate probabilities of superiority, arriving at conclusions such as “a randomly selected woman in the population of job applicants has 68% chance of having a higher empathy score than a randomly selected man” or “a randomly selected man in the population of job applicants has a 72% chance of having a higher math score than a randomly selected woman.”
If the hiring manager were to use this information to decide between the last two prospective employees, the hiring manager would commit to being wrong 32% of the time when hiring for empathy based on gender or wrong 28% of the time when hiring for math ability based on gender.
The given values for the probabilities are just examples, but this nonetheless illustrates why the category-based prejudice approach leads to a weaker conclusion than the direct measurement approach. The conclusion of the category-based prejudice approach is at best probabilistic and so will lead to decisions based on false beliefs for some percentage of the time in the long run.
These weaker, probabilistic conclusions can easily be replaced by stronger, more relevant information by simply applying the instrument of measurement directly to the final two prospective employees that the hiring manager is considering.
Individuals Not Randomly Selected
The idea of a randomly selected individual from a population is a useful construct when learning probability theory and statistics. However, in real life scenarios, one rarely ever encounters a randomly selected individual from a population. The final two prospective employees in these example scenarios illustrate this phenomenon.
The prospective employees have applied to this specific job posting, and they have gone through a vetting process that may have included interviews and other examinations. They are thus not randomly selected from the population of job applicants. Indeed, if the job posting specifically asked for applicants with empathy skills or with math skills, or if the interviewers were specifically looking for evidence of empathy skills or math skills, there is reason to believe the final two prospective employees might be unusual in these traits.
Another way of conceiving of this phenomenon is that the true population of interest in these scenarios is not the population of all job applicants to the company, but the population of job applicants to this specific job posting who have made it through all the vetting to become one of the two final prospective employees. This is likely too small of a population for any kind of inference about probability densities.
Ambiguous Categorization
Even if prior work has established a more general proposition such as “women are more empathic” or “men are better at math” for the relevant population of interest, and even if the hiring manager has accepted the probabilistic nature of applying this information to the two specific individuals being considered for the job, the fact that categorization is arbitrary can lead to ambiguous and contradictory conclusions when using the category-based prejudice approach.
An example comes from an experiment in social psychology regarding susceptibility to stereotyping. In order to frame their experiment, Shih, Pittinsky, and Ambady (1999) claimed that Asian women are the object of two contradictory stereotypes prevalent in the United States: that those categorized as “Asian” are good at math, and that those categorized as “women” are bad at math.
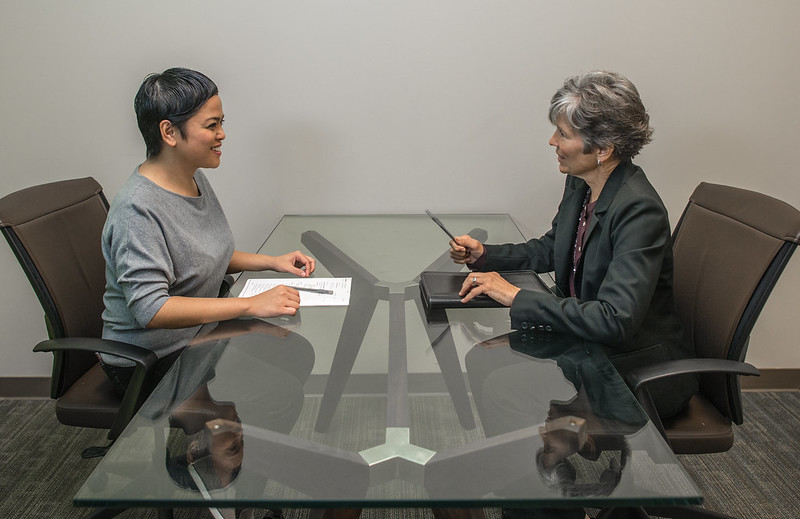
Suppose for the scenario in which the hiring manager is looking for math ability that both these stereotypes are prevalent,7 and that the woman among the two final prospective employees that the hiring manager is considering is categorized as “Asian,” while the man that the hiring manager is considered is not categorized as “Asian.” If the category-based prejudice approach were based on categorization by gender, it would lead to a greater probability of superiority in math skills for the man, but if it were based on categorization by Asian-ness, it would lead to a greater probability of superiority in math skills for the woman.
Because categorization is arbitrary, the hiring manager can pick whatever variable to use for the category-based prejudice approach. In cases in which the choice of two different variables, such as gender or Asian-ness, lead to different conclusions, the category-based prejudice approach leads to ambiguous results. In this scenario, category-based prejudice, in addition to its other flaws, does not actually resolve the problem it set out to solve in the first place: picking between the two final prospective employees.
Ambiguous Category Levels
As was seen in the abstract example, categorization is arbitrary not just in the choice of variable to use for categorization, but also in the choice of the levels of the variable.
This arbitrariness could be at play with regard to the rules for categorization as “Asian” in the scenario of hiring for math skills. For instance, the woman in the final two prospective employees could have a father with an Asian origin and a mother with origin outside of Asia. Indeed, because someone has 2n ancestors when considering n generations, it is conceivable that the woman could have an arbitrary percentage of ancestors of Asian origin and of non-Asian origin. This leads to the arbitrary choice of a cut point as discussed earlier, but in this case, with regard to how much Asian ancestry a particular person is required to have before the prejudice of “Asians are good at math” is to be applied.
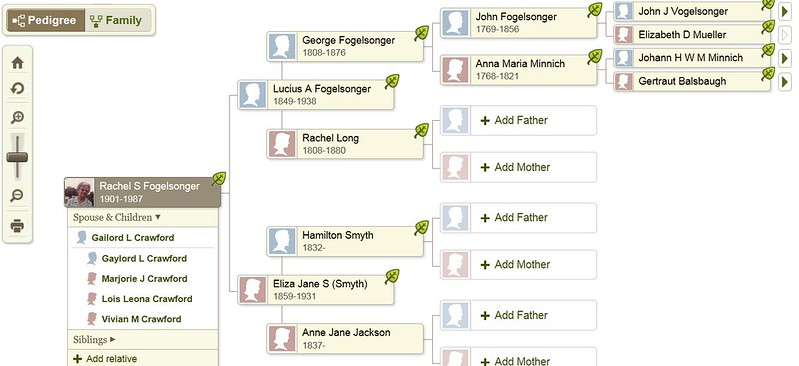
Furthermore, there are individuals who defy the levels of a categorical variable that are chosen to be used for category-based prejudice. For instance, suppose in the hiring scenarios instead of deciding between a man and a woman, the hiring manager is considering someone who has developed biologically intersexed and has not adopted any gender categorization. In such cases, it is not obvious how to apply the more general propositions such as “women are more empathic” or “men are better at math” for category-based prejudice, and the hiring manager would be left once more with an arbitrary choice of how to categorize the individual in order to use category-based prejudice.
Conclusion
This article extensively discusses flaws in using categorization, which might leave the reader with the impression that categorization is a bad thing. This is not the case. Categorization is a useful tool for understanding trends occurring in large populations. Like any useful tool, though, categorization can be abused. Because of this, it is important to discriminate between sound use of categorization and flawed abuse of categorization.
The particular kind of abuse of categorization that this article discusses occurs when instead of the analysis of trends in large populations, categorization is used in the judgment of individuals. Whenever categorization is used to judge individuals, the arguments outlined in this article apply, and it can be concluded that such use of categorization is fallacious.
While it is true that all instances of category-based prejudice are fallacies in the use of categorization, the converse is not the case: not all fallacies in the use of categorization are instances of category-based prejudice. This specific fallacy of categorization is merely the subject of this article. Other abuses of categorization, such as the arbitrary choice of cut point in order to effect the results of an analysis, are worthy topics in their own right and are discussed further elsewhere.
The main argument of this article is that judging individuals based on a general proposition about a population in which they are categorized is inferior to a better, alternative approach. In order for a general proposition about a population to be established, there must be some instrument of measurement used. This instrument of measurement can therefore be used for the direct measurement of the individuals. Such a direct measurement approach is less costly, more accurate, and less prone to ambiguities stemming from the arbitrariness of categorization than the use of category-based prejudice.
Citations
Footnotes
In particular, beta distributions were used throughout. Beta distributions are useful for generating example data because they are bounded, continuous, and can be parameterized to be symmetric or skewed as desired. The data set thus generated and used to create Figure 1 is available for download.↩︎
Because the physical dimensions of Figure 1 may be different depending on the device resolution used by the reader, abstract units of measurement are used such that both the height and the width of the two-dimensional plane are 10.↩︎
Alternatively, if the population is small and easily accessible, it might be more prudent to take a census of the entire population rather than sample from it.↩︎
In statistics, “power” has a specific mathematical meaning. It refers to one minus the Type II error rate. The Type II error rate is the probability of failing to reject the null hypothesis when the null hypothesis is indeed false. In statistical inference, drawing a conclusion is framed in terms of rejecting the null hypothesis. Therefore, Type II error rate measures how probable it is for a test to fail to draw a conclusion when a conclusion should have been drawn. Type II error rate can be thought of as the “false negative” rate. The lower the Type II error rate of a statistical test, the more power it has, and the more capable it is at inferring a conclusion.↩︎
The obvious exception would be a company in Massachusetts providing therapy services.↩︎
Point estimates such as the estimate of a population mean are insufficient to calculate a probability of superiority. Means are indicators of the central tendency of a distribution, which is just one aspect of the distribution. In order to calculate probability of superiority, one can assume or attempt to establish that the probability density of the variable of interest conforms to some established mathematically defined distributions for which there is a closed form solution for probability of superiority. Alternatively, one could estimate the density empirically, using techniques such as kernel density estimation.↩︎
Curiously, the authors report that the stereotype that Asians are good at math was more prevalent in the United States than in Canada based on a poll they did of random samples of people in Vancouver, British Columbia and in Cambridge, Massachusetts, but did not report just how prevalent the stereotype was in either place. (Why this is a bad practice is discussed extensively in the article on how to scrutinize a statistic. Indeed, it is used as an example in one section of said article.)
The authors cite a few other papers in their introduction when attempting to establish the premise that there are stereotypes that Asians are good at math and that women are bad at math, but these cited papers describe test scores, which is a different phenomenon from the prevalence of stereotypes.
A review of the literature finds numerous papers about the supposed effects of stereotypes on math performance, but few about the prevalence of such stereotypes. For the purposes of this article, the existence of these stereotypes only functions to motivate a thought experiment, and no claim is made as to how prevalent they are.↩︎